The pop glossary of Artificial Intelligence: meanings, context and trends
29 Jan 2025
4 min 53 sec
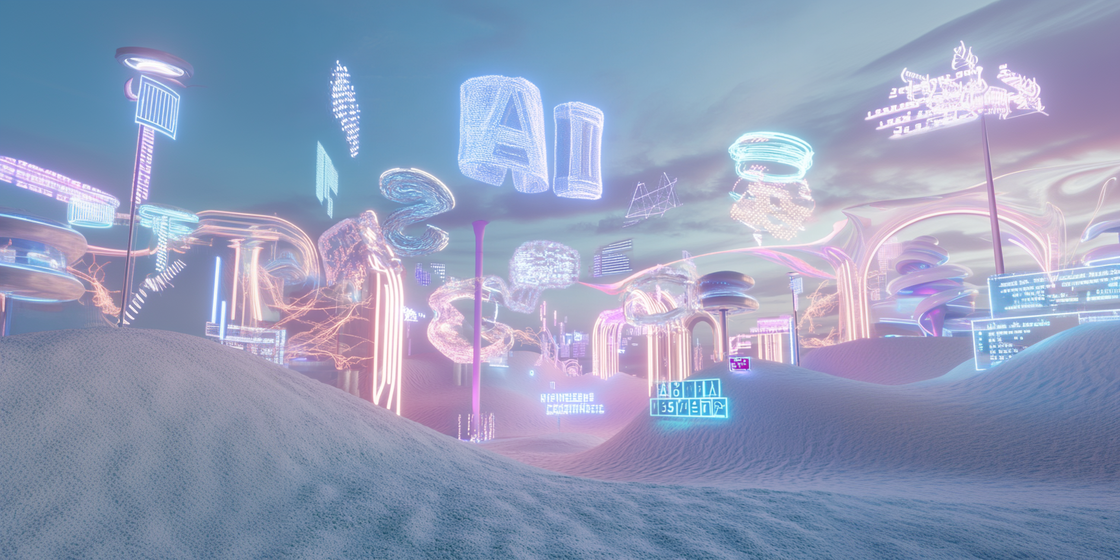
Artificial intelligence is redefining the way we work, communicate and make decisions, so much so that its impact is now impossible to ignore. With its spread, a number of technical terms have emerged and become commonly used, many of which have now transcended the world of specialists and entered the language of business and digital culture. Understanding this new vocabulary is not only useful for those working in tech, but also for those who want to understand how AI is shaping the future of businesses and professionals.
Machine Learning and Deep Learning
Machine learning is one of the fundamental concepts of artificial intelligence and refers to the ability of machines to learn from data without being explicitly programmed. The term was introduced in the 1950s by computer science pioneer Arthur Samuel, but it is with the increase in computational power and the availability of data that it has found its widespread application. Within machine learning, deep learning represents the most advanced evolution: models based on deep neural networks capable of processing huge amounts of data and recognizing complex patterns. It is thanks to deep learning that today we have systems such as facial recognition, self-driving cars, and generative models such as ChatGPT. In recent years, research on these two terms has grown exponentially, demonstrating their increasingly pervasive impact. If you whant to know more about ML algorithms read our article here.
Decision Intelligence
The concept of decision intelligence (here a deep dive article) emerged around 2018 and describes the use of artificial intelligence to support and optimize decision making. Unlike traditional data analytics, which provide only descriptive or predictive information, decision intelligence combines AI models, simulations, and business logic to help companies and organizations make faster and more effective decisions. This approach is particularly useful in areas such as risk management, marketing, and supply chain, where there are multiple and ever-changing variables to consider, as in WhAI platform. Since 2020, research on this term has increased by 150 percent, a sign that more and more organizations are adopting advanced tools to turn data into strategic actions.
Agentic AI
AI is moving from being a simple reactive tool to an autonomous entity capable of making decisions independently, and this is where the concept of agentic AI comes in . Appearing in discussions about the future of AI beginning in 2021, this term denotes intelligent systems that do not simply respond to external inputs, but act on defined goals and in a proactive manner. These AIs can analyze complex environments and perform actions without human intervention. The growing interest in proactive automation, from autonomous campaign management to artificial intelligence applied to robotics, has led to a 200 percent increase in Google searches related to this concept. Read our article on Agentic AI here.
Explainable AI (XAI)
With the increased use of artificial intelligence in critical areas such as finance, healthcare, and security, it has become essential to understand how and why an AI makes certain decisions. The concept of explainable AI (XAI) was born in 2016 with the launch of a project by DARPA, the U.S. Department of Defense Advanced Research Projects, with the goal of developing transparent and interpretable artificial intelligence models. The XAI (here our article about Explainable AI) allows users to understand the logic that drives an algorithm's decisions, reducing the risk of bias, error and discrimination. The topic of transparency in AI has become increasingly relevant, with research on this concept increasing by 180% in the past five years.
Natural Language Processing (NLP)
Natural language processing (NLP) is the discipline of enabling computers to understand, analyze, and generate human language. Although the first studies on this topic date back to the 1970s, the term gained popularity with the advent of chatbots and voice assistants. Today, NLP underlies technologies such as machine translation, semantic search engines and speech synthesis tools. Interest in this area has exploded with the launch of advanced models such as ChatGPT, Google Bard and Claude AI, growing NLP searches by 250% in recent years.
Slop
As the production of AI-generated content has increased, the term slop has taken on a new meaning. While it was initially used in technical forums to refer to inefficient code or dirty data, it is now increasingly being used to describe the phenomenon of the overproduction of homogenized content that lacks originality and real value. The web is increasingly flooded with automatically generated text, images and videos, often indistinguishable from each other and made without any creative insight or contribution. This phenomenon is raising questions about the quality of information and the future of content creation, with an open debate about how to maintain a balance between automation and human value.
Synthetic Data
One of the most emerging concepts in AI is that of synthetic data, or artificially generated data to train artificial intelligence models. The use of synthetic data has become increasingly relevant in recent years, especially to address issues related to privacy, scarcity of quality real-world data, and the need to balance datasets to avoid bias. Unlike data collected from the real world, synthetic data can be created to represent specific scenarios, allowing models to learn without ethical risks or data protection regulatory compliance issues. This approach is finding application in areas such as finance, healthcare, and autonomous driving, and research on this concept has grown by 170% in the past year.
Conclusion
The vocabulary of AI is growing as its applications evolve, and these terms represent only part of the transformation taking place. AI is no longer just a supporting technology, but is becoming a real player in the world of business and creativity. While tools such as decision intelligence and agentic AI are making machines increasingly autonomous, the concept of slop reminds us that not everything generated by AI has value. Understanding these concepts means not only staying up-to-date, but also being prepared to make the most of the opportunities presented by innovation.
'Decision Intelligence' saw a +150% increase in research since 2020: AI no longer just analyzes data, but drives decisions